
The proteome is the entire set of proteins that is, or can be, expressed by a genome, cell, tissue, or organism at a certain time. It is the set of expressed proteins in a given type of cell or organism, at a given time, under defined conditions. Proteomics is the study of the proteome.
Types of proteomes
While proteome generally refers to the proteome of an organism, multicellular organisms may have very different proteomes in different cells, hence it is important to distinguish proteomes in cells and organisms.
A cellular proteome is the collection of proteins found in a particular cell type under a particular set of environmental conditions such as exposure to hormone stimulation.
It can also be useful to consider an organism's complete proteome, which can be conceptualized as the complete set of proteins from all of the various cellular proteomes. This is very roughly the protein equivalent of the genome.
The term proteome has also been used to refer to the collection of proteins in certain sub-cellular systems, such as organelles. For instance, the mitochondrial proteome may consist of more than 3000 distinct proteins.[1][2][3]
The proteins in a virus can be called a viral proteome. Usually viral proteomes are predicted from the viral genome[4] but some attempts have been made to determine all the proteins expressed from a virus genome, i.e. the viral proteome.[5] More often, however, virus proteomics analyzes the changes of host proteins upon virus infection, so that in effect two proteomes (of virus and its host) are studied.[6]
Importance in cancer
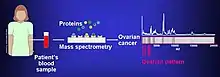
The proteome can be used in order to comparatively analyze different cancer cell lines. Proteomic studies have been used in order to identify the likelihood of metastasis in bladder cancer cell lines KK47 and YTS1 and were found to have 36 unregulated and 74 down regulated proteins.[7] The differences in protein expression can help identify novel cancer signaling mechanisms.
Biomarkers of cancer have been found by mass spectrometry based proteomic analyses. The use of proteomics or the study of the proteome is a step forward in personalized medicine to tailor drug cocktails to the patient's specific proteomic and genomic profile.[8] The analysis of ovarian cancer cell lines showed that putative biomarkers for ovarian cancer include "α-enolase (ENOA), elongation factor Tu, mitochondrial (EFTU), glyceraldehyde-3-phosphate dehydrogenase (G3P), stress-70 protein, mitochondrial (GRP75), apolipoprotein A-1 (APOA1), peroxiredoxin (PRDX2) and annexin A (ANXA)".[9]
Comparative proteomic analyses of 11 cell lines demonstrated the similarity between the metabolic processes of each cell line; 11,731 proteins were completely identified from this study. Housekeeping proteins tend to show greater variability between cell lines.[10]
Resistance to certain cancer drugs is still not well understood. Proteomic analysis has been used in order to identify proteins that may have anti-cancer drug properties, specifically for the colon cancer drug irinotecan.[11] Studies of adenocarcinoma cell line LoVo demonstrated that 8 proteins were unregulated and 7 proteins were down-regulated. Proteins that showed a differential expression were involved in processes such as transcription, apoptosis and cell proliferation/differentiation among others.
The proteome in bacterial systems
Proteomic analyses have been performed in different kinds of bacteria to assess their metabolic reactions to different conditions. For example, in bacteria such as Clostridium and Bacillus, proteomic analyses were used in order to investigate how different proteins help each of these bacteria spores germinate after a prolonged period of dormancy.[12] In order to better understand how to properly eliminate spores, proteomic analysis must be performed.
History
Marc Wilkins coined the term proteome [13] in 1994 in a symposium on "2D Electrophoresis: from protein maps to genomes" held in Siena in Italy. It appeared in print in 1995,[14] with the publication of part of his PhD thesis. Wilkins used the term to describe the entire complement of proteins expressed by a genome, cell, tissue or organism.
Size and contents
The genomes of viruses and prokaryotes encode a relatively well-defined proteome as each protein can be predicted with high confidence, based on its open reading frame (in viruses ranging from ~3 to ~1000, in bacteria ranging from about 500 proteins to about 10,000).[15] However, most protein prediction algorithms use certain cut-offs, such as 50 or 100 amino acids, so small proteins are often missed by such predictions.[16] In eukaryotes this becomes much more complicated as more than one protein can be produced from most genes due to alternative splicing (e.g. human proteome encodes about 20,000 proteins, but some estimates predicted 92,179 proteins out of which 71,173 are splicing variants).[17]
Association of proteome size with DNA repair capability
The concept of “proteomic constraint” is that DNA repair capacity is positively correlated with the information content of a genome, which, in turn, is approximately related to the size of the proteome.[18] In bacteria, archaea and DNA viruses, DNA repair capability is positively related to genome information content and to genome size.[18] “Proteomic constraint” proposes that modulators of mutation rates such as DNA repair genes are subject to selection pressure proportional to the amount of information in a genome.[18]
Proteoforms. There are different factors that can add variability to proteins. SAPs (single amino acid polymorphisms) and non-synonymous single nucleotide polymorphisms (nsSNPs) can lead to different "proteoforms"[19] or "proteomorphs". Recent estimates have found ~135,000 validated nonsynonymous cSNPs currently housed within SwissProt. In dbSNP, there are 4.7 million candidate cSNPs, yet only ~670,000 cSNPs have been validated in the 1,000-genomes set as nonsynonymous cSNPs that change the identity of an amino acid in a protein.[19]
Dark proteome. The term dark proteome coined by Perdigão and colleagues, defines regions of proteins that have no detectable sequence homology to other proteins of known three-dimensional structure and therefore cannot be modeled by homology. For 546,000 Swiss-Prot proteins, 44–54% of the proteome in eukaryotes and viruses was found to be "dark", compared with only ~14% in archaea and bacteria.[20]
Human proteome. Currently, several projects aim to map the human proteome, including the Human Proteome Map, ProteomicsDB, isoform.io, and The Human Proteome Project (HPP). Much like the human genome project, these projects seek to find and collect evidence for all predicted protein coding genes in the human genome. The Human Proteome Map currently (October 2020) claims 17,294 proteins and ProteomicsDB 15,479, using different criteria. On October 16, 2020, the HPP published a high-stringency blueprint [21] covering more than 90% of the predicted protein coding genes. Proteins are identified from a wide range of fetal and adult tissues and cell types, including hematopoietic cells.
Methods to study the proteome

Analyzing proteins proves to be more difficult than analyzing nucleic acid sequences. While there are only 4 nucleotides that make up DNA, there are at least 20 different amino acids that can make up a protein. Additionally, there is currently no known high throughput technology to make copies of a single protein. Numerous methods are available to study proteins, sets of proteins, or the whole proteome. In fact, proteins are often studied indirectly, e.g. using computational methods and analyses of genomes. Only a few examples are given below.
Separation techniques and electrophoresis
Proteomics, the study of the proteome, has largely been practiced through the separation of proteins by two dimensional gel electrophoresis. In the first dimension, the proteins are separated by isoelectric focusing, which resolves proteins on the basis of charge. In the second dimension, proteins are separated by molecular weight using SDS-PAGE. The gel is stained with Coomassie brilliant blue or silver to visualize the proteins. Spots on the gel are proteins that have migrated to specific locations.
Mass spectrometry
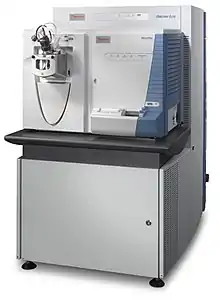
Mass spectrometry is one of the key methods to study the proteome.[22] Some important mass spectrometry methods include Orbitrap Mass Spectrometry, MALDI (Matrix Assisted Laser Desorption/Ionization), and ESI (Electrospray Ionization). Peptide mass fingerprinting identifies a protein by cleaving it into short peptides and then deduces the protein's identity by matching the observed peptide masses against a sequence database. Tandem mass spectrometry, on the other hand, can get sequence information from individual peptides by isolating them, colliding them with a non-reactive gas, and then cataloguing the fragment ions produced.[23]
In May 2014, a draft map of the human proteome was published in Nature.[24] This map was generated using high-resolution Fourier-transform mass spectrometry. This study profiled 30 histologically normal human samples resulting in the identification of proteins coded by 17,294 genes. This accounts for around 84% of the total annotated protein-coding genes.
Chromatography
Liquid chromatography is an important tool in the study of the proteome. It allows for very sensitive separation of different kinds of proteins based on their affinity for a matrix. Some newer methods for the separation and identification of proteins include the use of monolithic capillary columns, high temperature chromatography and capillary electrochromatography.[25]
Blotting
Western blotting can be used in order to quantify the abundance of certain proteins. By using antibodies specific to the protein of interest, it is possible to probe for the presence of specific proteins from a mixture of proteins.
Protein complementation assays and interaction screens
Protein-fragment complementation assays are often used to detect protein–protein interactions. The yeast two-hybrid assay is the most popular of them but there are numerous variations, both used in vitro and in vivo. Pull-down assays are a method to determine what kinds of proteins a protein interacts with.[26]
Protein structure prediction
Protein structure prediction can be used to provide three-dimensional protein structure predictions of whole proteomes. In 2022, a large-scale collaboration between EMBL-EBI and DeepMind provided predicted structures for over 200 million proteins from across the tree of life.[27] Smaller projects have also used protein structure prediction to help map the proteome of individual organisms, for example isoform.io provides coverage of multiple protein isoforms for over 20,000 genes in the human genome.[28]
Protein databases
The Human Protein Atlas contains information about the human proteins in cells, tissues, and organs. All the data in the knowledge resource is open access to allow scientists both in academia and industry to freely access the data for exploration of the human proteome. The organization ELIXIR has selected the protein atlas as a core resource due to its fundamental importance for a wider life science community.
The Plasma Proteome database contains information on 10,500 blood plasma proteins. Because the range in protein contents in plasma is very large, it is difficult to detect proteins that tend to be scarce when compared to abundant proteins. This is an analytical limit that may possibly be a barrier for the detections of proteins with ultra low concentrations.[29]
Databases such as neXtprot and UniProt are central resources for human proteomic data.
See also
References
- ↑ Johnson, D. T.; Harris, R. A.; French, S.; Blair, P. V.; You, J.; Bemis, K. G.; Wang, M.; Balaban, R. S. (2006). "Tissue heterogeneity of the mammalian mitochondrial proteome". American Journal of Physiology. Cell Physiology. 292 (2): c689–c697. doi:10.1152/ajpcell.00108.2006. PMID 16928776. S2CID 24412700.
- ↑ Morgenstern, Marcel; Stiller, Sebastian B.; Lübbert, Philipp; Peikert, Christian D.; Dannenmaier, Stefan; Drepper, Friedel; Weill, Uri; Höß, Philipp; Feuerstein, Reinhild; Gebert, Michael; Bohnert, Maria (June 2017). "Definition of a High-Confidence Mitochondrial Proteome at Quantitative Scale". Cell Reports. 19 (13): 2836–2852. doi:10.1016/j.celrep.2017.06.014. ISSN 2211-1247. PMC 5494306. PMID 28658629.
- ↑ Gómez-Serrano, M (November 2018). "Mitoproteomics: Tackling Mitochondrial Dysfunction in Human Disease". Oxid Med Cell Longev. 2018: 1435934. doi:10.1155/2018/1435934. PMC 6250043. PMID 30533169.
- ↑ Uetz, P. (2004-10-15). "From ORFeomes to Protein Interaction Maps in Viruses". Genome Research. 14 (10b): 2029–2033. doi:10.1101/gr.2583304. ISSN 1088-9051. PMID 15489322.
- ↑ Maxwell, Karen L.; Frappier, Lori (June 2007). "Viral proteomics". Microbiology and Molecular Biology Reviews. 71 (2): 398–411. doi:10.1128/MMBR.00042-06. ISSN 1092-2172. PMC 1899879. PMID 17554050.
- ↑ Viswanathan, Kasinath; Früh, Klaus (December 2007). "Viral proteomics: global evaluation of viruses and their interaction with the host". Expert Review of Proteomics. 4 (6): 815–829. doi:10.1586/14789450.4.6.815. ISSN 1744-8387. PMID 18067418. S2CID 25742649.
- ↑ Yang, Ganglong; Xu, Zhipeng; Lu, Wei; Li, Xiang; Sun, Chengwen; Guo, Jia; Xue, Peng; Guan, Feng (2015-07-31). "Quantitative Analysis of Differential Proteome Expression in Bladder Cancer vs. Normal Bladder Cells Using SILAC Method". PLOS ONE. 10 (7): e0134727. Bibcode:2015PLoSO..1034727Y. doi:10.1371/journal.pone.0134727. ISSN 1932-6203. PMC 4521931. PMID 26230496.
- ↑ An, Yao; Zhou, Li; Huang, Zhao; Nice, Edouard C.; Zhang, Haiyuan; Huang, Canhua (2019-05-04). "Molecular insights into cancer drug resistance from a proteomics perspective". Expert Review of Proteomics. 16 (5): 413–429. doi:10.1080/14789450.2019.1601561. ISSN 1478-9450. PMID 30925852. S2CID 88474614.
- ↑ Cruz, Isa N.; Coley, Helen M.; Kramer, Holger B.; Madhuri, Thumuluru Kavitah; Safuwan, Nur a. M.; Angelino, Ana Rita; Yang, Min (2017-01-01). "Proteomics Analysis of Ovarian Cancer Cell Lines and Tissues Reveals Drug Resistance-associated Proteins". Cancer Genomics & Proteomics. 14 (1): 35–51. doi:10.21873/cgp.20017. ISSN 1109-6535. PMC 5267499. PMID 28031236.
- ↑ Geiger, Tamar; Wehner, Anja; Schaab, Christoph; Cox, Juergen; Mann, Matthias (March 2012). "Comparative Proteomic Analysis of Eleven Common Cell Lines Reveals Ubiquitous but Varying Expression of Most Proteins". Molecular & Cellular Proteomics. 11 (3): M111.014050. doi:10.1074/mcp.M111.014050. ISSN 1535-9476. PMC 3316730. PMID 22278370.
- ↑ Peng, Xing-Chen; Gong, Feng-Ming; Wei, Meng; Chen, Xi; Chen, Ye; Cheng, Ke; Gao, Feng; Xu, Feng; Bi, Feng; Liu, Ji-Yan (December 2010). "Proteomic analysis of cell lines to identify the irinotecan resistance proteins". Journal of Biosciences. 35 (4): 557–564. doi:10.1007/s12038-010-0064-9. ISSN 0250-5991. PMID 21289438. S2CID 6082637.
- ↑ Chen, Yan; Barat, Bidisha; Ray, W. Keith; Helm, Richard F.; Melville, Stephen B.; Popham, David L. (2019-03-15). "Membrane Proteomes and Ion Transporters in Bacillus anthracis and Bacillus subtilis Dormant and Germinating Spores". Journal of Bacteriology. 201 (6). doi:10.1128/JB.00662-18. ISSN 0021-9193. PMC 6398275. PMID 30602489.
- ↑ Wilkins, Marc (Dec 2009). "Proteomics data mining". Expert Review of Proteomics. England. 6 (6): 599–603. doi:10.1586/epr.09.81. PMID 19929606. S2CID 207211912.
- ↑ Wasinger VC, Cordwell SJ, Cerpa-Poljak A, Yan JX, Gooley AA, Wilkins MR, Duncan MW, Harris R, Williams KL, Humphery-Smith I (1995). "Progress with gene-product mapping of the Mollicutes: Mycoplasma genitalium". Electrophoresis. 16 (1): 1090–94. doi:10.1002/elps.11501601185. PMID 7498152. S2CID 9269742.
- ↑ Kozlowski, LP (26 October 2016). "Proteome-pI: proteome isoelectric point database". Nucleic Acids Research. 45 (D1): D1112–D1116. doi:10.1093/nar/gkw978. PMC 5210655. PMID 27789699.
- ↑ Leslie, Mitch (2019-10-18). "Outsize impact". Science. 366 (6463): 296–299. Bibcode:2019Sci...366..296L. doi:10.1126/science.366.6463.296. ISSN 0036-8075. PMID 31624194. S2CID 204774732.
- ↑ Uniprot, Consortium (2014). "UniProt: a hub for protein information". Nucleic Acids Research. 43 (D1): D204–D212. doi:10.1093/nar/gku989. ISSN 0305-1048. PMC 4384041. PMID 25348405.
- 1 2 3 Acosta S, Carela M, Garcia-Gonzalez A, Gines M, Vicens L, Cruet R, Massey SE. DNA Repair Is Associated with Information Content in Bacteria, Archaea, and DNA Viruses. J Hered. 2015 Sep-Oct;106(5):644-59. doi: 10.1093/jhered/esv055. Epub 2015 Aug 29. PMID: 26320243
- 1 2 Aebersold, Ruedi; Agar, Jeffrey N; Amster, I Jonathan; Baker, Mark S; Bertozzi, Carolyn R; Boja, Emily S; Costello, Catherine E; Cravatt, Benjamin F; Fenselau, Catherine; Garcia, Benjamin A; Ge, Ying (March 2018). "How many human proteoforms are there?". Nature Chemical Biology. 14 (3): 206–214. doi:10.1038/nchembio.2576. hdl:1721.1/120977. ISSN 1552-4450. PMC 5837046. PMID 29443976.
- ↑ Perdigão, Nelson; et al. (2015). "Unexpected features of the dark proteome". PNAS. 112 (52): 15898–15903. Bibcode:2015PNAS..11215898P. doi:10.1073/pnas.1508380112. PMC 4702990. PMID 26578815.
- ↑ Adhikari, S (October 2020). "A high-stringency blueprint of the human proteome". Nature Communications. 11 (1): 5301. Bibcode:2020NatCo..11.5301A. doi:10.1038/s41467-020-19045-9. PMC 7568584. PMID 33067450.
- ↑ Altelaar, AF; Munoz, J; Heck, AJ (January 2013). "Next-generation proteomics: towards an integrative view of proteome dynamics". Nature Reviews Genetics. 14 (1): 35–48. doi:10.1038/nrg3356. PMID 23207911. S2CID 10248311.
- ↑ Wilhelm, Mathias; Schlegl, Judith; Hahne, Hannes; Gholami, Amin Moghaddas; Lieberenz, Marcus; Savitski, Mikhail M.; Ziegler, Emanuel; Butzmann, Lars; Gessulat, Siegfried; Marx, Harald; Mathieson, Toby; Lemeer, Simone; Schnatbaum, Karsten; Reimer, Ulf; Wenschuh, Holger; Mollenhauer, Martin; Slotta-Huspenina, Julia; Boese, Joos-Hendrik; Bantscheff, Marcus; Gerstmair, Anja; Faerber, Franz; Kuster, Bernhard (2014). "Mass-Spectrometry-Based Draft of the Human Proteome". Nature. 509 (7502): 582–7. Bibcode:2014Natur.509..582W. doi:10.1038/nature13319. PMID 24870543. S2CID 4467721.
- ↑ Kim, Min-Sik; et al. (May 2014). "A draft map of the human proteome". Nature. 509 (7502): 575–81. Bibcode:2014Natur.509..575K. doi:10.1038/nature13302. PMC 4403737. PMID 24870542.
- ↑ Shi, Yang; Xiang, Rong; Horváth, Csaba; Wilkins, James A. (2004-10-22). "The role of liquid chromatography in proteomics". Journal of Chromatography A. Bioanalytical Chemistry: Perspectives and Recent Advances with Recognition of Barry L. Karger. 1053 (1): 27–36. doi:10.1016/j.chroma.2004.07.044. ISSN 0021-9673. PMID 15543969.
- ↑ "Pull-Down Assays - US". www.thermofisher.com. Retrieved 2019-12-05.
- ↑ Callaway, Ewen (2022-07-28). "'The entire protein universe': AI predicts shape of nearly every known protein". Nature. 608 (7921): 15–16. Bibcode:2022Natur.608...15C. doi:10.1038/d41586-022-02083-2. PMID 35902752. S2CID 251159714.
- ↑ Sommer, Markus J.; Cha, Sooyoung; Varabyou, Ales; Rincon, Natalia; Park, Sukhwan; Minkin, Ilia; Pertea, Mihaela; Steinegger, Martin; Salzberg, Steven L. (2022-12-15). "Structure-guided isoform identification for the human transcriptome". eLife. 11: e82556. doi:10.7554/eLife.82556. PMC 9812405. PMID 36519529.
- ↑ Ponomarenko, Elena A.; Poverennaya, Ekaterina V.; Ilgisonis, Ekaterina V.; Pyatnitskiy, Mikhail A.; Kopylov, Arthur T.; Zgoda, Victor G.; Lisitsa, Andrey V.; Archakov, Alexander I. (2016). "The Size of the Human Proteome: The Width and Depth". International Journal of Analytical Chemistry. 2016: 7436849. doi:10.1155/2016/7436849. ISSN 1687-8760. PMC 4889822. PMID 27298622.
External links
- PIR database
- UniProt database
- Pfam database at the Library of Congress Web Archives (archived 2011-05-06)